Connecting Data and Life to Achieve a Healthy Future.

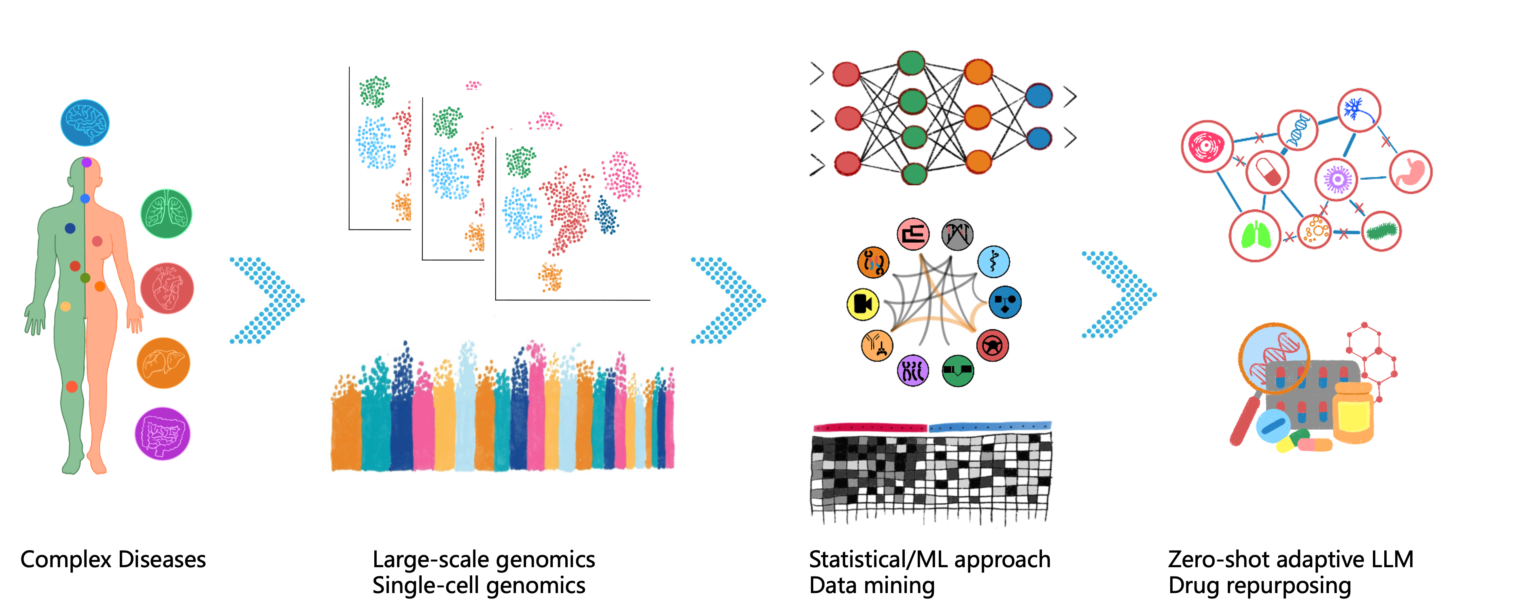
- Our research focuses on exploring the molecular genetic mechanisms of complex diseases through the development of statistical genetics methods, large-scale genomics, single-cell genomics, and multi-omics data analysis.
- We are active in the cutting-edge fields of computational, statistical, and machine learning method development and application. These methods utilize large-scale and multi-omics data to understand the occurrence, progression, and treatment responses of complex diseases (such as pulmonary fibrosis, cardiovascular diseases) and to explore corresponding treatment strategies.
- We advocate for proactive interdisciplinary collaboration, dedicated to solving real-world biological and genomic translational genomics problems. We welcome experts from various disciplines, including biologists, computational biologists, bioinformaticians, computer scientists, clinical medicine specialists, immunologists, and oncologists, to collaborate with us.
Research Interests
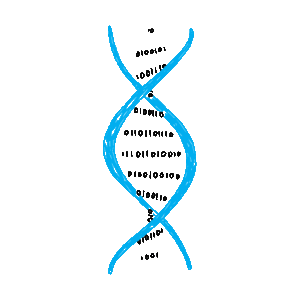
Statistical Genetics and Functional Genomics
Human genetic variants are crucial natural probes in studying the cellular and gene regulation associated with complex human diseases. A large number of existing Genome-Wide Association Studies (GWAS) have identified many genetic variant loci associated with susceptibility to complex diseases. What are the molecular mechanisms behind these associated loci? Which cell types mediate the gene regulation of corresponding disease characteristics? By developing new statistical genetics methods and combining GWAS results with functional genomics multi-omics, we further investigate the genetic variations, gene expression regulation, and the mechanisms of disease onset and progression in organisms.
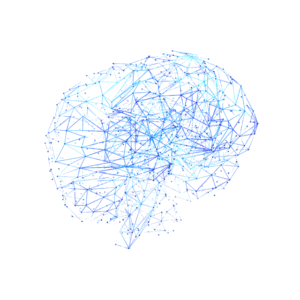
Machine Learning and Drug Discovery
Machine learning plays an essential role in handling the ever-increasing high-throughput biological data. We develop and utilize various cutting-edge machine learning methods and tools to provide effective and efficient solutions for biomedical research. Our main research directions include using machine learning to identify new drug targets and to repurpose existing drugs. These methods not only help us rapidly screen for potential candidate drugs but also aid in understanding the mechanisms of action of new drugs from a molecular genetics perspective.
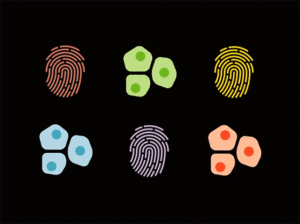
Single-cell Atlases for Complex Diseases
We focus on developing and improving a range of computational methods. These methods not only utilize machine learning models and large-scale single-cell genomic data but also include various types of omics data such as spatial transcriptomics and multi-omics. We are particularly interested in revealing the heterogeneity and dynamic changes of cells in complex diseases (such as pulmonary fibrosis, cardiovascular diseases, etc.) by establishing single-cell atlases of these diseases. Additionally, we are committed to enhancing the efficiency and accuracy of data processing through low-dimensional methods and interpretable deep learning models. Through these advanced analyses, we hope to gain a deeper understanding of the interactions between cells and how they play a role in the pathogenesis of complex diseases, thereby providing new strategies and directions for the treatment of these diseases.
Innovation, Collaboration, Challenge – if you have a strong passion for cutting-edge exploration in genomics and precisionmedicine, and also wish to work and grow in a healthy, thriving team, we warmly welcome you to join us.